Forms a border around an image.
C++:
Python:
C:
Python:
The function copies the source image into the middle of the destination image. The areas to the left, to the right, above and below the copied source image will be filled with extrapolated pixels. This is not what FilterEngine or filtering functions based on it do (they extrapolate pixels on-fly), but what other more complex functions, including your own, may do to simplify image boundary handling.
The function supports the mode when src is already in the middle of dst . In this case, the function does not copy src itself but simply constructs the border, for example:
Note: When the source image is a part (ROI) of a bigger image, the function will try to use the pixels outside of the ROI to form a border. To disable this feature and always do extrapolation, as if src was not a ROI, use borderType | BORDER_ISOLATED.
Reference: OpenCV Documentation - copyMakeBorder
Example
This is a sample code (C++) with images for opencv copyMakeBorder.
Download complete Visual Studio project.
Continue Reading...
C++:
void copyMakeBorder(InputArray src, OutputArray dst, int top, int bottom, int left, int right, int borderType, const Scalar& value=Scalar() )
Python:
cv2.copyMakeBorder(src, top, bottom, left, right, borderType[, dst[, value]]) → dst
C:
void cvCopyMakeBorder(const CvArr* src, CvArr* dst, CvPoint offset, int bordertype, CvScalar value=cvScalarAll(0) )
Python:
cv.CopyMakeBorder(src, dst, offset, bordertype, value=(0, 0, 0, 0)) → None
Parameters: |
|
The function copies the source image into the middle of the destination image. The areas to the left, to the right, above and below the copied source image will be filled with extrapolated pixels. This is not what FilterEngine or filtering functions based on it do (they extrapolate pixels on-fly), but what other more complex functions, including your own, may do to simplify image boundary handling.
The function supports the mode when src is already in the middle of dst . In this case, the function does not copy src itself but simply constructs the border, for example:
// let border be the same in all directions
int border=2;
// constructs a larger image to fit both the image and the border
Mat gray_buf(rgb.rows + border*2, rgb.cols + border*2, rgb.depth());
// select the middle part of it w/o copying data
Mat gray(gray_canvas, Rect(border, border, rgb.cols, rgb.rows));
// convert image from RGB to grayscale
cvtColor(rgb, gray, CV_RGB2GRAY);
// form a border in-place
copyMakeBorder(gray, gray_buf, border, border,
border, border, BORDER_REPLICATE);
// now do some custom filtering ...
...
Note: When the source image is a part (ROI) of a bigger image, the function will try to use the pixels outside of the ROI to form a border. To disable this feature and always do extrapolation, as if src was not a ROI, use borderType | BORDER_ISOLATED.
Reference: OpenCV Documentation - copyMakeBorder
Example
This is a sample code (C++) with images for opencv copyMakeBorder.
string imgFileName = "lena.jpg";
cv::Mat src = cv::imread(imgFileName);
if (!src.data){
cout << "Unable to open file" << endl;
getchar();
return 1;
}
Mat dstBorderConstant;
copyMakeBorder(src, dstBorderConstant, 256, 256, 256, 256, BORDER_CONSTANT);
Mat dstBorderDefault;
copyMakeBorder(src, dstBorderDefault, 256, 256, 256, 256, BORDER_DEFAULT);
Mat dstBorderIsolate;
copyMakeBorder(src, dstBorderIsolate, 256, 256, 256, 256, BORDER_ISOLATED);
Mat dstBorderReflect;
copyMakeBorder(src, dstBorderReflect, 256, 256, 256, 256, BORDER_REFLECT);
Mat dstBorderReflect101;
copyMakeBorder(src, dstBorderReflect101, 256, 256, 256, 256, BORDER_REFLECT101);
Mat dstBorderReflect_101;
copyMakeBorder(src, dstBorderReflect_101, 256, 256, 256, 256, BORDER_REFLECT_101);
Mat dstBorderReplicate;
copyMakeBorder(src, dstBorderReplicate, 256, 256, 256, 256, BORDER_REPLICATE);
Mat dstBorderWrap;
copyMakeBorder(src, dstBorderWrap, 256, 256, 256, 256, BORDER_WRAP);
cv::namedWindow("Source", CV_WINDOW_FREERATIO);
cv::namedWindow("BorderConstant", CV_WINDOW_FREERATIO);
cv::namedWindow("BorderDefault", CV_WINDOW_FREERATIO);
cv::namedWindow("BorderIsolate", CV_WINDOW_FREERATIO);
cv::namedWindow("BorderReflect", CV_WINDOW_FREERATIO);
cv::namedWindow("BorderReflect101", CV_WINDOW_FREERATIO);
cv::namedWindow("BorderReflect_101", CV_WINDOW_FREERATIO);
cv::namedWindow("BorderReplicate", CV_WINDOW_FREERATIO);
cv::namedWindow("BorderWrap", CV_WINDOW_FREERATIO);
cv::imshow("Source", src);
cv::imshow("BorderConstant", dstBorderConstant);
cv::imshow("BorderDefault", dstBorderDefault);
cv::imshow("BorderIsolate", dstBorderIsolate);
cv::imshow("BorderReflect", dstBorderReflect);
cv::imshow("BorderReflect101", dstBorderReflect101);
cv::imshow("BorderReflect_101", dstBorderReflect_101);
cv::imshow("BorderReplicate", dstBorderReplicate);
cv::imshow("BorderWrap", dstBorderWrap);
cv::waitKey(0);
cv::imwrite("BorderConstant.jpg", dstBorderConstant);
cv::imwrite("BorderDefault.jpg", dstBorderDefault);
cv::imwrite("BorderIsolate.jpg", dstBorderIsolate);
cv::imwrite("BorderReflect.jpg", dstBorderReflect);
cv::imwrite("BorderReflect101.jpg", dstBorderReflect101);
cv::imwrite("BorderReflect_101.jpg", dstBorderReflect_101);
cv::imwrite("BorderReplicate.jpg", dstBorderReplicate);
cv::imwrite("BorderWrap.jpg", dstBorderWrap);
Filtered Image | Source Image |
borderType - BORDER_REFLECT borderType - BORDER_REFLECT101 borderType - BORDER_REFLECT_101 borderType - BORDER_REPLICATE borderType - BORDER_CONSTANT borderType - BORDER_DEFAULT borderType - BORDER_ISOLATED borderType - BORDER_WRAP |
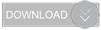
Download complete Visual Studio project.